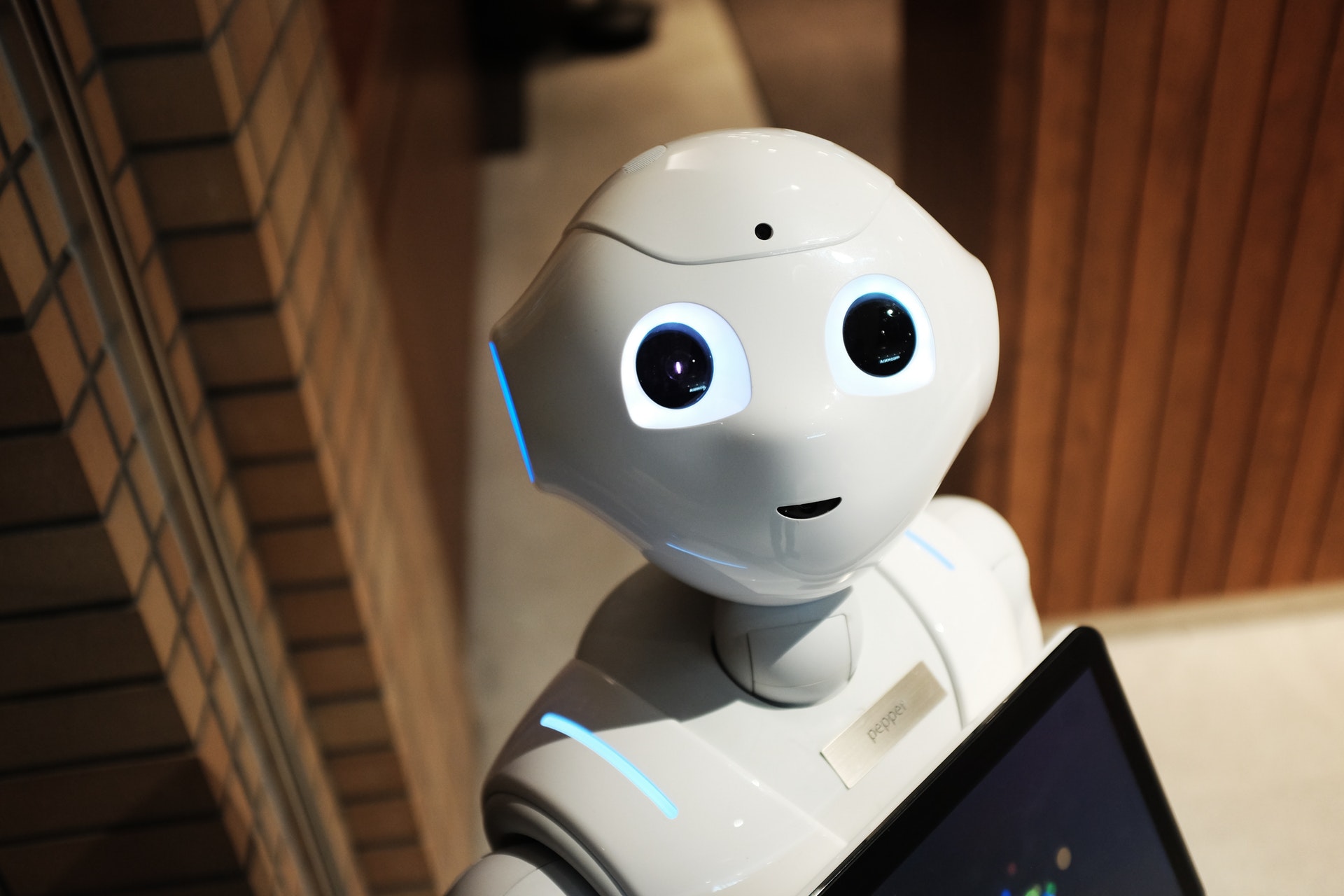
Anshul Jain
27 May 2020
In the recent few years, the advancement in technology has found a new foreground. Every year, we come across a breakthrough in technology that revolutionizes industries and promises to change the way we live and work. Be it 3D Printing, Artificial Embryos, Blockchain, Data Science, or any other tech, technology has progressed in ways no one could have expected.
These trends of tomorrow are already stirring our economy and society, with smart businesses and countries already making plans to invest and capitalize from them.
“A report from the Global Institute estimates that Big Data could generate an additional $3 trillion in value every year in just seven industries.”
This offers clarity to the fact that these innovations of today will continue to grow and have a major impact on the world in the years ahead, as they will open a new door for creativity and innovation, which will further allow people to experiment with new ideas.
However, the focus here is not on the possibilities of innovation in technology, but to discuss a technology that stands the tallest today and has the potential to not only change our lives but make it futuristic.
Artificial Intelligence (AI) with its analytical and human-like qualities is giving way to computing systems that can see, hear, learn, and reason, which will help create opportunities to improve education and healthcare, address poverty, and achieve a more sustainable future.
But, that’s not all!
AI is bound by no limitations yet and will be explored viciously by the experts of all industries.
So, let us try and understand the various trends affiliated to this technology and recognize what stands in the future of Artificial Intelligence and how will it change the “normal life”.
Introduction to Artificial Intelligence: The Next Era of Computing
“Artificial intelligence would be the ultimate version of Google. The ultimate search engine that would understand everything on the web. It would understand exactly what you wanted, and it would give you the right thing...” - Larry Page
Artificial Intelligence or AI, as it is commonly known, found its origin way back in 1956, but its popularity has only reached its zenith today because of increased data volumes, advanced algorithms, as well as improvements in computing power and storage.
However, why is Artificial Intelligence suddenly so popular? What makes it unique and unconventional that organizations around the world are eager to invest their money in it?
If we go by the statistics, Artificial Intelligence is considered to be the next big thing to rule the world. As stated by PwC in their Global Artificial Intelligence Study “AI could contribute up to $15.7 trillion to the global economy in 2030, more than the current output of China and India combined.” Moreover, it “can transform the productivity and GDP potential” of the same.
So, what makes it this important?
This can simply be answered by understanding what Artificial Intelligence is?
Defining Artificial Intelligence(AI):
Artificial Intelligence is defined by different individuals/experts differently. However, John McCarthy, the Father of Artificial Intelligence, who coined the term in 1956, defines it as “the science and engineering of making intelligent machines.” It is based on this definition that AI found its modern meaning. Though the difference in definitions is still there, their underlying concept is the same.
Hence,
AI or Machine Intelligence is a technology that makes it possible for a machine to learn from experience, adjust to inputs, and perform human-like tasks and functions, without major human interference. It is, in short, the intelligence demonstrated by machines, which is at par with the natural intelligence displayed by us humans as well as animals.
For example,
A Chess Game on a computer has the ability to beat its human opponent. This portrayal of human-like thinking is the oldest form of artificial intelligence accessible to us human beings.
However, there is a limitation associated with this older version of AI, as it can only perform functions that it is programmed to do. That is, though this computer is capable of defeating its human opponent in the chess game, it lacks the ability to solve any other complex equation or problem on its own. Hence, it relies heavily on the programming done by humans/engineers.
As all current AI is narrow/weak (a type of AI) or dependent on problem-solving algorithms designed by programmers, experts nowadays are eager to overcome this shortcoming in modern Artificial Intelligence, by creating various AI algorithms that are a combination of machine learning and different data analysis methods , which allows AI systems to be independent and devoid of any human interference and perform tasks commonly associated with intelligent beings.
Now that we understand the basics of AI let us delve deeper into it and give a look at its various types.
Levels of Artificial Intelligence(AI)
Before we discuss the types of AI, it is crucial we understand its various levels. Artificial Intelligence is mainly categorized into two major levels, Weak AI/Narrow AI and Strong AI/Artificial General Intelligence (AGI), however, there is another level of AI that is still unexplored, but that has a great virtual presence, i.e. Super Intelligence.
-
Weak AI/Narrow AI:
Weak or Narrow AI refers to computers/machines/systems that are focused on one narrow task, which they are trained/programmed to perform. Currently, the AI-powered intelligent machines we are most familiar with are mainly Narrow.
For example, Apple’s Siri, NVIDIA Self Driving Car, IBM’s Watson are all Narrow or Weak AI.
-
Strong AI/Artificial General Intelligence (AGI):
Strong AI or Artificial General Intelligence (AGI) is the holy grail of AI, as it has the capability to learn, solve, and perform any task that it is presented, without any human intervention.
Applications and computers powered by Strong AI have the same accuracy level as humans, as they are endowed with generalized human-like cognitive abilities, such as adaptability, thinking, learning, creating, deciding, etc.
Currently, there hasn’t been any major developments in the field of AGI, but researchers around the world are invested in accomplishing this dream soon, with large-scale organizations investing their money to support it.
-
Superintelligence:
This is the third level of AI, which is the future potential creation of AGI. Superintelligence will be a result of recursive self-improvement, which is considered to be a major global catastrophic risk by the intellectuals of our time, as it will be surpassing the brightest of human minds.
As said by Stephen Hawking“The development of full artificial intelligence could spell the end of the human race."
Though researchers are miles away from making SuperIntelligence a reality, its concept is already a favorite of storytellers around the world. Movies like The Terminator; The Matrix; I, Robot; among others, constantly deal with the idea of Super Intelligence, which is usually represented as being apocalyptic and catastrophic for humans.
It is not yet clear where the end of this tale of Artificial Intelligence will be, but denying the possibility of it being destructive for the future generations shouldn’t be overruled, as even the great minds like Elon Musk consider it as an ‘existential risk’.
Types of Artificial Intelligence(AI):
There are basically four (4) types of Artificial Intelligence, as categorized by Arend Hintze, the Assistant Professor at Michigan State University.
-
Reactive Machines:
WThe first as well as the most basic layer of Artificial Intelligence, Reactive Machines do not have the ability to form memories, neither do they use past experiences to inform current decisions.
Machines designed with a Reactive system and intelligence perceive the world directly and act on what they encounter or see. They do not rely on any internal concept of the world, nor do they use elaborated learning algorithms to improve their perception.
Example:
A Reactive Machine example that is commonly used to define this concept is IBM’s famous Supercomputer Deep Blue that won against the Russian Chess Grandmaster, Garry Kasparov, in 1997, using its ability to simply analyze possible moves, both of its own and its opponents, and choose the most strategic one.
The idea behind Deep Blue was to narrow its view to stop it from pursuing future moves, based on how it rated its outcomes. Without this ability, it would have been difficult for Deep Blue to defeat Kasparov. Google’s AlphaGo is another Reactive Machine that is designed for narrow purposes and that cannot be applied to other situations.
These methods though improve the ability of AI systems in certain specified areas or situations, they, however, can’t be easily changed or applied to any other situation. Moreover, as they lack the concept of a wider world, these can’t interactively participate in the world, the way we imagine AI systems to do and can be easily fooled.
In short, machines and systems with Reactive Machine AI continue to behave in a similar manner every time they encounter a similar situation.
-
Limited Memory:
Machines that have the ability to look into past experiences to inform future decisions, use the technology of Limited Memory, which is the second layer of Artificial Intelligence types.
Example:
Self Driving Cars are one such example that consists of a few features that use this AI technology. Leveraging Limited Memory, these cars observe and store data such as the recent speed of the nearby car, its distance, speed limits, lane markings, traffic lights and other important elements, like curves in the road help it navigate roads without any issue.
These observations are added to the cars' preprogrammed representations of the world, which are used in situations like when the car change lanes or tries to avoid cutting off another driver or being hit by a nearby car.
With limited memory, self-driving cars are capable of making decisions based on past experiences, yet they are unable to retain the data for a long period of time, which makes it transient, as it is not saved in the car's library.
-
Theory of Mind:
Theory of Mind is the AI of the future and is still far from being achievable, though researchers are deeply invested in turning it into a reality.
This technology is based on the psychological concept of theory of mind, which refers to the understanding that others have their own beliefs, desires, and intentions that impact their decision.
Researchers hope that machines developed with the concept of theory of mind will be able to imitate human-like mental models, by forming representations about the world, as well as about other agents and entities in it.
Moreover, it will be able to relate to humans and perceive human intelligence and emotions, while understanding how it is affected by events and the environment.
-
Self-Awareness:
The fourth layer and the last type of Artificial Intelligence, Super-Awareness, like Theory of Mind is limited to sci-fi and is yet to be a reality, but researchers believe it to be the ultimate goal of development of intelligent machines computer science.
Machines with self-awareness will have a sense of self or “consciousness” and they will be able to infer their current state and use that information to further understand what other (humans or machines) are feeling.
This, in a way, can be termed as an extension of the concept of Theory of Mind, the third level of AI, as like the latter, it too deals with the idea of AI using its ability to understand its surroundings, with the assistance of various machine learning algorithms, data sets, deep learning,etc.
Hence, the types of Artificial Intelligence can, therefore, be categorized into two sets,
-
1. Where Reactive Machines and Limited Memory, the existing AI, are grouped together and
-
2. Where Theory of Mind and Self-Awareness, the non-existing AI, are grouped together.
Artificial Intelligence(AI) Global Impact
“A year spent on Artificial Intelligence is enough to make one believe in God”- Alan Perlis, Computer Scientist & Professor at Purdue University
The last few years have been most prosperous for artificial intelligence and its various branches, with it gaining a renowned global recognition and importance, which has made it the center of economic attention. Moreover, AI is primed to pervade everyday life in the form of various autonomous vehicles to intelligent chatbots, apps, ads, and more.
However, this recognition and significance of AI, gives way to an acute question, as to what impact will it have globally and how will it change the socio-economic trends of the world.
Well, the answer to this question is not yet clear, but the current technological trends, stats, and data highlight the future of AI’s global impact and how it will change the way of our lives. Moreover, the way countries around the world are adopting this new tech also becomes a bridge to calculating the universal influence of AI.
So, let us try and answer this question as well as get an understanding of how it is being used globally.
Global Growth:
In today's world, where Siri and Alexa are an integral part of our day-to-day lives, it is not surprising to see that the global impact of AI is monumental and not limited to just one industry, country, or sector.
As stated by McKinsey Global Institute 2010, the global funding for AI startups has grown at an outstanding 60% compound annual growth rate, which is bound to increase within the upcoming years.
Moreover, with different communities around the world actively discussing the influence and possibilities AI will exert over both global corporations and national strength, there is no doubt the shift to automation and AI will be soon enough and it will introduce us to an era which will be just futuristic. As stated by Microsoft’s chief envisioner David Coplin “It will change how we relate to each other. I would argue that it will even change how we perceive what it means to be human.”
So, though new, where is the impact of AI most evident?
The impact of Artificial Intelligence is already transformative and monumental across all sectors and industries. It is with its assistance that organizations around the world are able to perform tasks that were earlier impossible or extremely difficult.
From helping transportation companies predict arrival times or various critical issues to predicting toxins in the food grains, the impact that AI is having on the world is endless. Moreover, it has offered scientists the ability to explore and learn how to treat cancer more effectively and allowed farmers to figure out how to grow more food using fewer natural resources.
With its level of ease and convenience, Artificial Intelligence (AI) has opened new doors for businesses globally, due to which the number of organizations that have adopted AI in some way or another has risen from 25% in 2018 to 37% in January 2019, as informed by the global market research firm Gartner.
Though most of AI’s economic impact is yet to come, its development has tripled within a year, with 52% of telecom organizations deploying chatbots and 38% of healthcare providers relying on computer-assisted diagnostics.
Moreover, this development is not merely restricted to industries like telecom, retail, or healthcare. There are various underlying and fundamental technologies such as behavioral algorithms, suggestive searches like social media feeds, and autonomously-powered self-driving vehicles that are boasting powerful and predictive capabilities of Artificial Intelligence.
While extremely useful, all of these are mostly basic AI and are yet to have a great change. However, with companies like Apple, Facebook, Google, and Tesla rolling out new ground-breaking updates, the impact these AI driven technologies will have on their audience is going to increase dramatically in the coming years.
Hence, there is no doubt that AI is and will continue to be the key source of transformation, disruption, and competitive advantage in today’s fast-changing economy.
Let us further understand the impact of AI by looking at the countries leading in AI.
Impact that Artificial Intelligence has On Leading Countries:
The impact that artificial intelligence has on countries around the world varies depending on numerous aspects. However, there is no denying the fact that AI has had an effect on the socio-economic status of several countries, which has further resulted in an increase in the overall global economy.
As calculated by a 2017 study done by PWC, the global GDP will be 14 percent higher by 2030 as a result of AI adoption, contributing an additional $15.7 trillion to the global economy.
Ever since the future of Artificial Intelligence started appearing brighter, countries like Canada, China, Denmark, India, Italy, Japan, Mexico, Singapore, South Korea, Sweden, Taiwan, the European Commission, Finland, France, the UAE, the UK among others, have all released official strategies to promote the use and development of AI.
In fact, there are countries like the United Arab Emirates that have recently appointed their first minister responsible for AI.
It is, though, difficult to qualify and categorize success and leadership in the context of AI. It can be categorized with the assistance of the following points:
- a) The availability of the talent: Countries that already have a pool of qualified and learned AI experts are leveraging the benefits of this technology.
- b) Level of AI & Digital Activity in the country: These are the countries that are building the foundation necessary to support the future of AI, as they have the necessary amount of funding in circulation, AI-adjacent companies in operation and patents being granted.
It is based on these two major points that we will categories the countries currently leading in the development/investment of AI, which are:
-
1. China:
Currently leading the realm of AI and AI research, China is the first country to host a major AI International Standards Meeting. With most effective and comprehensive AI strategies and plans, compared to other nations, China’s State Council’s promise to become a $150 billion AI Global leader by 2030, can be a realistic and achievable one.
Moreover, the country's interest in AI can be measured through the investments it made in AI startups. According to CB Insights, Chinese Startups received 48% of funding for all AI-related investments.
-
2. United States:
The United States of America is the second leading country in AI. However, there is not a major investment in the realm of technology, but it is being led by academia and private industry. Moreover, there are no policies associated with AI in the USA, but individual projects, funded by military and paramilitary departments like DARPA and IARPA are helping the country achieve success in the field of artificial intelligence.
-
3. Japan:
Japan, a country which is well known for its innovations and technology, is the third leading country in AI. Like China, Japan has also created a council to focus on the development and commercialization of AI.
The focus of the country is mainly on developing research and development (R&D) goals and to create a roadmap for industrialization of artificial intelligence.
-
4. United Kingdom:
The United Kingdom is a leader in AI in Europe and the fourth leading country in the world, with 121 AI firms. The tech companies in the UK raised private investment of a total of $8.6 billion in 2017, which amounts to 38 percent of all venture capital invested there.
-
5. European Union:
European Union, too, holds a high ambition for AI. As recommended by European Commission, the executive branch of the European Union, the EU is planning to drastically increase investments in AI, which will help them maximize the impact of investments at EU and national levels and encourage synergies and cooperation.
Moreover, a report from the European Commission (April 25th2018) outlines a $24 billion (€20 billion) investment between 2018 and 2020, with the expectation that those funds will come from public and private entities.
-
6. Germany:
Germany, like China, plans to become a leading hub for artificial intelligence. It is among those countries that have the most number of published research papers on AI, which is estimated to be near 8,000 between 2011-2015.
These researches are not the only indicator of investment in AI, as there are various fields–related citations, that define the state of AI in the country. As reported by the FT article, many technical universities and leading companies like Porsche, Bosh, Daimler, etc. are combining their knowledge of AI to welcome the future in Germany. The Cyber Valley is also playing a major role in this merger, as companies like Amazon are planning to open an AI research lab there.
-
7. France:
With 120 AI enterprises and a plan to open and host government data as part of its AI Strategy, France is the 7th leading country in AI. The country’s AI initiative is highly focused on data, as it hopes to make private companies publicly release their data for use in AI on a case-by-case basis.
Moreover, as announced by President Emmanuel Macron at the end of AI for Humanity Summit in Paris (2018), the French government will invest $1.8 billion (€1.5 billion) in AI research until 2022, to transform into a global leader in AI research, training, and industry.
-
8. Canada:
The first country to release a national AI Strategy, Canada has a five (5) year plan to invest, as detailed in the 2017 budget, an outstanding amount of $ 125 million in AI research and talent.
The country has cities like Toronto, Montreal, and Edmonton that are home to some of the world's finest AI degree programs, which are helping new talents grow in the field of AI and opening doors for companies like Facebook to invest in Canada to access that talent.
-
9. Russia:
Though there are no formal policies in place for artificial intelligence in Russia, the country is enthusiastically researching AI. The country spends an estimated $12.5 million annually on AI, with its plan to create a state system for AI education and talent retainment establishing a national center for AI, and hosting war games to study the impact of AI on military operations.
The country though is invested in complete AI implementation, its major focus in AI is military in nature, as it plans to make the military and equipment robotic and artificially intelligent by 2030.
-
10. Sweden:
With 55 AI enterprises, Sweden is the 10th country that has been affected by the revolutionary advancement in Artificial Intelligence. Though this impact is not drastic and clear, the country is optimistic and is working with Nordic-Baltic regions to implement AI in their society.
-
11. India:
Another important Asian country on the list, India, is taking all the necessary steps to implement Artificial Intelligence to improve its economic growth as well as use it for social inclusion.
This shift towards artificial intelligence in India is coming from companies that make up its $143 billion outsourcing industry. Firms like Infosys, TCS, and Wipro are providing services to huge names like Pepsi, Kellogg, etc. who are rapidly adopting AI to automate their operations, which is helping India leverage AI for economic growth and social inclusion.
-
12. Israel:
Israel is another important country that is being affected by the global growth of Artificial Intelligence. Though the country lacks any formal AI policy, its influence there is not lacking at all. With its AI startups tripling since 2014, Israel has already embarked on the largest AI to exist to date and boasts a substantial and growing cluster of startups utilizing and developing AI technologies.
As reported by Hackernoon, “Israel’s AI startup ecosystem has raised over $7.5 billion cumulatively.”
Currently, the US and China are dominating the realm of AI because of their different and unique approaches. These countries have the necessary infrastructure, innovations, and the initiatives that help them leverage the benefits of AI.
Moreover, the ways in which other countries are investing in this technology reflects that though, the advancement of Artificial Intelligence is still in its infancy, there is no doubt that its upcoming future will be full of new innovations that will further highlight its capabilities and may even take it to its next level.
Industry:
In the section above, we listed some countries that are currently leading in the research and development of AI. While classifying the global investment in AI, what we realized is that the research in AI is not just associated with or limited to one industry. From IT and telecom industries to manufacturing, financial, and more, its reach and impact can be felt everywhere.
However, there is a common misconception around the world that it takes a long time for AI to create an impact, but, whether we realize it or not, AI is indeed creating a rapid and global impact.
Every time we open our Facebook newsfeed, do a Google search, book a trip online, or get a product recommendation from our favorite e-commerce websites like Amazon, AI is lurking in the background, performing necessary assessments and delivering us suggestions that match our requirements.
There is no doubt that AI is set to be the key source of transformation and disruption in today’s age of smart factories and the Internet of Things (IoT) and it delivers a competitive advantage that manufacturers around the world cannot afford to ignore.
Therefore, there is a rising tide of AI adoption across industries that will drive significant growth in the next decade, with software revenue set to reach almost $90 billion by 2025. This collaboration of machines and humans will help industries better outputs and high-quality products, with less wastage of resources.
Now, that we understand the impact AI has on industries, let us zoom in more and identify the sectors/industries that rely heavily on Artificial Intelligence.
Industries Relying on Artificial Intelligence(AI):
1. Healthcare:
The impact of AI is tremendous in the field of healthcare, as it has opened new doors for diagnosing rare diseases. Moreover, it has offered researchers the ability to find suitable treatments, because of the accurate assessments made by it.
For example, IBM's cognitive supercomputer used its ability to perform assessments to diagnose a patient with a rare type of leukemia, which the doctors were unable to find even after months.
In short, AI is already a game-changer in the healthcare sector with its ability to analyze complex medical data, such as X-rays, CT Scans, etc. However, the involvement of AI, in this industry, is only limited to just being an aid rather than being a replacement for human physicians.
It is augmenting a physician’s diagnosis, which is in the process of helping AI gain valuable insight into various important treatments and assessments and learn and improve continuously.
2. Automotive:
The impact of AI on the automotive industry is the most prosperous one, as companies like Google, Waymo, and Tesla are already investing plenty of their resources and money to create cars with futuristic AI-powered features, like automatic braking, collision avoidance systems, pedestrians and cyclist alerts, cross-traffic alerts, intelligent cruise control, among others.
Moreover, traditional auto industry players like BMW, Ford, Toyota, and VW are using
AI as a critical component in their autonomous vehicle development to improve their capabilities and to make transportation more secure.
Hence, AI and its technologies are making self-driving cars secure and hassle-free for travelers. Moreover, AI trends like the Internet of Things (IoT), Cloud Computing, Machine Learning, Deep Learning, Infotainment Systems, and Cognitive Capabilities are further driving the automotive industry and helping it deliver excellence.
Considering this popularity, it is not shocking to see that the installation rate of AI-based systems in new vehicles is expected to rise to 109% in 2025.
In the coming years, this popularity of AI in the automotive industry will increase rapidly, as it will offer automobile makers the transparency they strive for. With AI embedded in their development process, the industry will have an idea of how the vehicles are made and how their inventory is being managed, which will help them improve their quality control process.
However, the impact of AI in the automotive industry is not limited to self-driving cars. Soon, it will be adopted in various other machines that will help us stay connected, on schedule, as well as safe, even when we are not behind the wheel or driving.
3. Finance & Financial services:
At the core of the financial sector lies the aspects for which intelligent machines are already famous. Accuracy, real-time reporting, and processing high volumes of quantitative data, all are areas that make AI and intelligent machines what they are.
Taking note of AI's efficiency and accuracy the financial industry is rapidly implementing various AI-powered technologies to simplify the costly and time-consuming process of human financial services and advice.
The biggest advantage of AI’s influence on the industry is that it has personalized financial planning and made detecting fraudulent transactions a reality. Now systems can use deep learning technologies and analyze patterns and spot suspicious behavior and potential fraud. In short, AI has paved the way for safer and more secure online transactions by bringing financial cybersecurity to the next level.
PayPal, for instance, has already made its mark in the industry by implementing AI and deep learning into their systems and reducing their fraud rate to just 0.3% of revenue.
This is made possible by enabling the systems to analyze transactions in real-time and depict a pattern that reflects suspicious activities.
However, that's not all Robo-Advisors are another gift that Artificial Intelligence gave to this industry. It is with the assistance of these automated portfolio managers that the industry is able to develop customized investment solutions for mass-market consumers.
Artificial intelligence hence has offered the advantage to manage finances dynamically to match the goals of the masses and optimize the client’s available funds.
4. Retail and E-Commerce:
The impact of AI is most evident in the Retail and E-Commerce industry, with new applications and software being invented to redefine the retail and consumer relationship. As reported by CB Insights, from 2013-18, AI startups raised $1.8 billion across 374 deals.
This remarkable growth in the industry is mainly a result of Amazon’s investment in AI and its technology. The company adopted AI in its processes and forced retailers to reconsider both the e-commerce and physical strategies to stay connected with their customers.
Moreover, with the introduction of Amazon Go in selective states of the US (Seattle, Chicago, & San Francisco) Amazon started a new trend of cashier-less shops and delivered customers a new ‘grab and go’ retail model, which is bound to expand globally.
So, how is AI changing the game in the retail and e-commerce industry?
The Retail and E-Commerce industry is trying to create a cohesive customer experience by implementing AI into various e-commerce practices. AI and AI algorithms are being used by companies to accumulate personal shopping trends, which are used to provide suitable suggestions to the customers.
Other ways in which AI is being used in the industry are:
- To create a customer-centric search: This is made possible by the recommendation engines that use machine learning to perform predictive analysis and provide personalized recommendations to the customers.
- To create a new level of personalization across multiple devices: With new and innovative advances in AI and machine learning, it is now possible to use them to further personalize retail & e-commerce services across multiple devices. Organizations are using AI algorithms to personalize the user’s experience, as it helps them build a personal relationship with customers, which further increases sales as well as create loyal and lasting relationships.
- To deploy chatbots: Companies are implementing AI to create conversation intelligent software like chatbots, which enable them to interact with customers and bring a personal touch to the entire exchange. It also enables them to follow up leads, by analyzing and segmenting calls, using speech recognition and natural language processing.
- To empower store members: Another recent use of AI in the industry is to empower store members so that they can deliver optimum customer services. Bossa Nova Robot used by Walmart is one such example that has become a familiar sight in grocery stores in the US. This safe and user-friendly robot is used to stack shelves and to keep track of the items and where they are located.
- To make delivery driver-less: Though still a plan in process, soon AI will be used to pilot autonomous delivery. Kroger and Nuro have partnered together to redefine the grocery customer experience and make the convenience of grocery delivery accessible and affordable for customers everywhere.
Though we have mentioned only a few uses of AI in the Retail & E-commerce industry, this list is non-exhaustive, as it is increasing day-by-day. And we are bound to come across most of them soon.
5. Technology, Media, & Telecommunications (TMT):
Technology, media, and telecommunications (TMT) sector, along with Artificial Intelligence, can efficiently lead companies, consumers, and the public sector into a digital world, as their investment in the technology, is the most thriving.
TMT companies, when compared with other industries, are spending extensively on AI technologies and getting the highest returns, that is, an ROI above 20%, as reported by Deloitte Insights.
Moreover, AI is being deployed into their business processes which is helping them deliver personalized recommendations within the entertainment sector as well as automating customer support or network operations in the telecom industry.
Technologies powered by AI and Machine Learning have already started transforming various industrial landscapes. Tech companies like Amazon, Google, IBM, and Microsoft are providing Cloud-based infrastructures that are responsible for powering the data and analytics revolution. Moreover, they are allowing businesses to create new opportunities around artificial intelligence.
Even the entertainment and media giants like Netflix, Spotify, and iQiyi, its counterpart in China, are using AI to enhance quality and improve the user experience. Whereas, Telecommunication companies (Safaricom and NTT Docomo) are offering digital services to consumers, such as improved financial inclusion in emerging markets, health care, commerce, financial, and lifestyle services, etc.
AI, in short, is offering the TMT companies the opportunity to reimagine their business model and generate revenue by selling AI-based services to other organizations that are still lagging behind in this venture.
6. Manufacturing:
From delivering significant cuts in unplanned downtime to a better-designed product, Artificial Intelligence’s (AI) deployment in the manufacturing industry is allowing enhanced monitoring and autocorrection, as well as enabling manufacturers to apply AI-powered analysis to data to improve efficiency, product quality, and the safety of the employees.
`
Moreover, AI’s self-learning abilities have made the manufacturing process more predictable and controllable, which is helping manufacturers reduce costly delays in production and deviations from product specifications. AI has made possible for manufacturers to create robot-driven assembly lines and intelligent systems that can predict the malfunctioning of the machinery. Other ways in which AI is helping the Manufacturing Industry are:
- Human-robot collaboration
- Implementation of Quality 4.0
- Product development with Generative Design
- Solving supply-chain concerns across all geographies.
Hence, there is no doubt that in the coming years, AI would become inevitable for the manufacturing industry. As reported by the company ARK in 2017, the price of industrial robots will drop by 65% by 2025, due to its extensive use and implementation in the manufacturing industry.
7. Transport:
Self-driving cars are though still a thing of the future for the general public, smart cars have already found their place in the market. Ever since 2015, the rate of implementation of AI-driven applications and systems in vehicles has risen and is bound to increase further 109% by 2025.
AI, therefore, is rapidly being adopted by companies and is being applied to increase efficiency and create new value in the industry. Organizations like Tesla, Daimler, Uber, etc. are coming together with startups to develop various autonomous vehicles like trucks, cars, etc. for professional uses. Apart from these, companies like Amazon are implementing AI into vehicles to automate delivery.
In short, from monitoring drivers to identifying & predicting potential cross traffic dangers, AI-driven technologies like cameras, navigation, auto-braking features, etc. are being used to improve driving experiences as well as to make it safe for travelers.
Hence, there is no doubt that AI is becoming a mega-trend in the travel and transportation industry and revolutionizing the way we travel and make travel arrangements, identify routes, among other things.
8. Logistics & Supply Chain:
Artificial Intelligence (AI) is already gaining traction in the commercial space and and is now picking up momentum in the logistics and supply chain industry, with its precision and ever-evolving nature, which are an important asset for this industry to distribute status quo and usual processes, which involved relentless focus and labor in all the operations.
As reported by BI Intelligence, “Supply chain and operations is the third most active area for the use of AI, with 42 percent of respondents seeing revenue gains from investments in the technology.” Also, a recent survey done by McKinsey global revealed that 76% of supply chain companies around the world have already reported a moderate to a significant increase in value and revenue from deploying AI into their processes.
The supply chain managers are using the capabilities of AI to analyze and track data, detect anomalies, and generate predictions to improve as well as connect the entire supply chain, from first to last.
Moreover, it is also optimizing delivery routes, reducing costs, maximizing speed, spurring innovation, as well as enhancing productivity across the entire supply chain. Hence, its implementation in the industry has made the entire process of supply chain management more streamlined and accurate, as it is with the assistance of AI that the global logistics and supply chain operations are able to manage and simplify the process of managing fleets of vehicles and networks of facilities worldwide.
From AI-enabled robots like inventory taking drones to automated vehicles like driverless warehouse carts, AI has and is powering many applications in the supply chain and logistics industry.
The industry is leveraging AI technologies like anticipatory logistics and machine learning, to develop advanced applications, which makes them capable of handling the immense volume of data generated by a typical supply chain.
For example, Natural Language Processing helps computers understand and interact with human speech with the assistance of artificial intelligence and machine learning, by reducing administrative overhead in the supply chain. Additionally, the popular shipping and logistics company DHL is also using AI and computer vision to improve their processes.
Other trends that are accelerating the use of AI in supply chain and logistics are:
- Computing Power
- Big Data
- Algorithm Advancements
Looking at the impact Artificial Intelligence and its technologies has had on various industries, it is not surprising to see Governments, tech giants, universities, are already a part of the game and are making use of this new technology to gain huge competitive advantages as well as to increase their review.
In short, AI along with machine learning, data analytics, automation, and deep learning is ready to transform the international trade and become the fourth major industrial revolution that will deliver new and advanced opportunities to the businesses and change the way the companies perform.
Artificial Intelligence(AI) Technologies to Lookout for:
Throughout our discussion, we have frequently mentioned technologies associated with Artificial Intelligence (AI). Whether it is machine learning, data analytics, image recognition, or any other tech, all of them are an integral part of AI and play a vital role in making it what it is.
So, let us now shift our focus on these technologies and understand their basics and how they change will and are impacting the socio-economic development.
1. Deep Learning Theory/Platforms:
A subset of Machine Learning, Deep Learning deals with algorithms inspired by the structure and function of the brain called artificial neural networks (ANN). These mirror the functioning of the human brain, process data, and create data for decision making.
This AI tech performs feature extractions by itself and is mainly used to recognize patterns and to classify applications compatible with large scale data sets.
2. Capsule Networks:
A machine learning system and a type of artificial neural network (ANN), Capsule Networks or Capsule Neural Networks process vital information like a brain, that is, it is able to maintain hierarchical relationships.
Capsule Networks is used to model these hierarchical relationships, as it has a neural net architecture that has a profound impact on deep learning, especially for computer vision. Moreover, it emulates the brain's visual processing strength and promises better accuracy via the reduction of errors for typical identification tasks.
3. Deep Reinforcement Learning:
Another type of neural network, Deep Reinforcement Learning (DRL) helps scale problems that were earlier impossible. This network learns by interacting with the environment through observations, actions, and rewards.
DRL is the most general learning technique that requires lesser data than other methods used for training data models. It makes use of deep learning and reinforcement learning principles to create efficient algorithms that can be applied to robotics, video games, finance, healthcare, etc.
4. Generative Adversarial Network:
Generative Adversarial Network or GAN is a type of unsupervised machine learning system that is implemented as two competing neural networks. It belongs to a set of generative models that are capable of producing new content through their ability to mimic any distribution of data.
GAN can be taught to create a world similar to ours in any domain, such as images, music, speech, prose. Moreover, it is recently being applied by various organizations like Facebook, in numerous fields to visualize interior/industrial design for computer games, to age face photographs, develop model patterns of motion in the video, reconstruct 3D models, etc.
5. Lean & Augmented Data Learning:
An important part of Artificial Intelligence, Lean and Augmented data learning is the ability of an application to learn through synthesized data, where the real historical data is unavailable.
Lean and augmented data learning is a regulator that prevents overfitting in neural networks and helps improve the performance of the imbalanced class problem. Moreover, it addresses the major challenge of labeled data.
6. Probabilistic Programming:
Probabilistic Programming or Probabilistic Programming Language (PPL) is a high-level programming language that helps developers design and automatically solve probability models, without any hassle. This language was specifically designed to describe and infer with Probabilistic Rational Models (PRMs) and is rapidly gaining traction in machine learning, AI, cognitive science, robotics, statistics, etc.
Moreover, a concept of PPL, probabilistic reasoning is used by companies like Google, Amazon, and Microsoft for various predicting stock prices, suggesting movies and shows, among other things.
7. Hybrid Learning Models:
A model designed to enhance the benefits of various other deep neural networks and models like GANs or DRL, Hybrid Learning Model combines two approaches to leverage the strengths of each.
With its assistance, one can achieve better performance and explainability of models, by expanding the variety of business problems to include deep learning. Bayesian Deep Learning, Bayesian GANs, etc. are few of the examples of Hybrid Learning Models.
8. Automated Machine Learning:
Automated Machine Learning or AutoML is the process of automating the time consuming and expert-driven workflow of developing machine learning models using a number of statistical and deep learning techniques.
It makes it easier for organizations to build and use machine learning models in the real world. Moreover, AutoML incorporates the best practices of machine learning to make data science more accessible for organizations.
9. Digital Twin or AI Modeling:
Digital Twins are digital replicas of physical or psychological systems that are used by data scientists to run simulations before actual systems are built and deployed. It is an important concept of the Internet of Things (IoT) and is rapidly changing the ways in which technologies like IoT, Artificial Intelligence, and Analytics are optimized.
Moreover, this virtual model is used to facilitate detailed analysis and monitoring of physical and psychological systems. From the industrial sector to more, this technology is being incorporated by tech giants like Tesla and Volkswagen to their business processes.
10. Explainable AI:
A contrast to the scores of machine learning algorithms in use today that can think, sense, and act differently in numerous applications, Explainable AI is a movement that aims to develop machine learning techniques that produce more explainable models while maintaining their prediction accuracy.
Also known as Interpretable Artificial Intelligence or Transparent Artificial Intelligence, Explainable AI, in short, is hoping to gain an insight into the “black boxes” of these algorithms to increase their adaptation across sectors and to establish trust.
11. Robotic Processes Automation or Workforce:
The process of using software with artificial intelligence and machine learning capabilities to perform and handle high- volumes and repeatable tasks that require intensive labor is known as Robotic Processes Automation.
It mimics and automates human tasks to support corporate operations and is mainly used in situations where it is too expensive or inefficient to get human experts. Blue Prism, WorkFusion, UiPath are some of the companies using this technology in their day to day business processes.
12. Natural Language Processing:
Natural Language Processing (NLP) is a technology that enables a software application to recognize, interpret, and synthesize speech or human language, as it is spoken. It is a subfield of Artificial Intelligence and is focused on making communicating with computers and systems understandable and easy.
Leveraging the abilities like machine learning and statistical methods, it uses text analytics to comprehend sentences, their structure, meaning, as well as intent. NLP is currently in boom and can be found in various Virtual Assistants, like Google Assistant & Microsoft’s Cortana, Apple’s Siri, Amazon’s Alexa, etc.
13. Natural Language Generation:
A sub-discipline of Artificial Intelligence, Natural Language Generation (NLG) converts a variety of data into human-readable text, which enables computers and systems to rapidly communicate ideas, with perfect accuracy.
This technology is related to AI concerned with a human to machine and contrariwise, like Natural Language Processing (NLP), Computation Linguistics, etc. Moreover, it is adopted by organizations that are eager to transform their business and innovate on how they better engage with their customers.
14. Speech Recognition:
Also known as automatic speech recognition or computer speed recognition, Speech Recognition is the ability of software or computer to identify words and phrases in any spoken language and to convert them into a machine-readable format.
It works using algorithms through acoustic and language modeling and is implemented in system control, navigation, commercial or industrial applications, as well as voice dialing.
15. Voice Assistance:
A technology powered by Natural Language Processing (NLP), Voice Assistance or Intelligence Personal Assistant, facilitates the transcription and transformation of human speech into a comprehensible form for software or computer.
Voice Assistance performs tasks for an individual based on verbal commands and is another popular technology used by organizations like Apple, Google, Microsoft, Samsung, etc. for voice dialing, voice search, call routing, speech-to-text processing, among others.
16. Virtual Agents:
Virtual Agents are computer programs or agents that are capable of interacting with humans in a human-like manner. These understand customer’s intent and can provide them with personalized answers, such as Siri, Cortana, Alexa, chatbots, or any other advanced system.
Virtual Agents, therefore, are able to make intelligent conversation and can respond to queries and work 24/7. Organizations currently scaling in the implementation of this technology are IBM, Google, Apple, etc.
17. Machine Learning Platforms:
Machine Learning and its platforms are the current trendsetters, as they enable computers and software to sieve through and analyze large sets of data and identify as well as predict useful patterns. It makes intelligent machines that can teach themselves and improve from data without any external interference or programming.
Moreover, machine learning platforms consist of various algorithms, development tools, APIs, model deployment, etc. that help companies like Microsoft, Amazon, and Google, improvise and improve their business processes.
18. AI Optimized Hardware:
AI optimized hardware has sped the next-generation of applications and given way to new graphics and central processing units. It has made possible for developers to create devices with integrated AI, chips, and graphics processing units (GPUs) that are tailored to execute AI-oriented tasks.
These appliances will bring about the next level of gaming, while simultaneously propelling the deep learning. Moreover, it will deliver the best average sustained performance possible and enable organizations like Intel, IBM, Google, Nvidia, etc. to develop machines that depict certain aspects of human intelligence, such as learning, reasoning, and self-correction.
19. Decision Management:
Like hardware, companies are also integrating artificial intelligence with decision management to leverage its capabilities like quick assessment and delivering quality data. Artificial Intelligence has automated decision management and made it more reliable and efficient.
It is with the assistance of AI that organizations are able to make consistent, efficient and information-driven decisions. Moreover, it is helping decision management systems in translating customer data into predictive models of key trends.
20. Decision Support System:
Decision Support System (DSS) is an informational application or a computer program application that analyzes business data, which is further used to support decision making in an organization. It has access to large volumes of information that is generated from various related information systems.
It is with the assistance of DSS that one can make more informed decisions, perform timely problem solving, and improve the efficiency of dealing with problems.
21. Biometrics and Authentication:
Biometrics and Authentication is the latest technology that is making use of AI to improve its processes. It is a security process that enables natural interaction between humans and machines, including interactions related to touch, image, speech, and body language recognition.
Commonly used in market research and security reasons, it is mainly concerned about the metrics related to human characteristics and is used to manage access to physical and digital resources such as buildings, rooms, etc.
22. Computer Vision:
The process of analyzing and understanding visual content and information is a complex task that requires artificial intelligence. It is with the assistance of computer vision that this complexity is reduced.
Computer vision uses machines to understand and analyze images and visual content. It is concerned with its automatic extraction and analysis. Additionally, it develops a theoretical and algorithmic basis that is used to achieve automatic visual understanding as well as to produce numeric or symbolic information.
23. Artificial Creativity:
Also known as Computational Creativity or Mechanical Creativity, Artificial Creativity is a multidisciplinary endeavor that is located at the intersection of artificial intelligence, cognitive psychology, arts, and philosophy.
Designed with the aim to model, stimulate, and replicate creativity using a machine or computer, it can help one to effortlessly achieve one of several ends. Moreover, it is mainly concerned with theoretical and practical issues in the study of creativity.
24. Computer Automated Design:
An extension of Computer-Aided Design, Computer Automated Design (CAD) is the use of computers or machines for the creation, modification, analysis, as well as optimization of a design. This technique enables organizations to focus on high-level design, rather than the mechanics of the drawing technology.
CAD is helping designers eliminate repetitive tasks, increase designing speed and consistency, and is simplifying condensed processes. Moreover, organizations are able to decrease engineering time through rules-based computer automated design systems and automated configurations.
25. Cyber Defense:
Like other areas, integration of artificial intelligence, along with Machine Learning, is recently evident in cyber defense. Leveraging the flexibility and learning capabilities of AI, companies like Darktrace are using it to detect online enemies and to defend against the dynamically as well as puissant cyber attacks.
It is because of this integration that appliances are able to pick up subtle indicators of abnormal activities and perform response actions like self-patching.
26. Compliance:
To ensure that a person or organization meets the requirements of accepted rules, legislation, rules, etc. a confirmation is done by the authority. However, recently this too is leveraging the use of AI and machine learning to enhance the compliance team’s view of monitored users, help them identify compliance-related communication, as well as to ensure that is implemented properly, which further facilitates analyst’s decision making.
In short, AI and machine learning with their capacity to reliably analyze data, offer teams and organizations the ability to quickly quarantine suspicious activity, in real-time.
27. Knowledge Worker Aid:
Artificial Intelligence is vastly helping employees in their work and processes, especially those who are in knowledge work. Every day, companies around the world are using the technology in the area to improve the quality of various diagnoses and assessments.
Moreover, medical and legal professionals are rapidly adopting AI to aid knowledge workers in their tasks, so they can get better assessments and results. Kim Technologies and Kyndi are two organizations that are currently working towards making knowledge workers aid a regular thing.
28. Content Creation:
Leveraging its capabilities like machine learning, image recognition and natural language processing (NLP), Artificial Intelligence is now capable of creating content. From reviews, and articles to the product description and more, it is delivering content that is believable and useful.
It is also helping organizations predict what messages and images will drive desired consumer action and response. A few of the brands that are already using AI to generate content are USA Today, Hearst, and CBS.
29. Peer-to-Peer Networks:
Artificial Intelligence and Blockchain are revolutionizing the peer-to-peer (P2P) networks, making it more secure and reliable. It is being used by organizations around the world to create transparency in various processes as well as to enhance their services and applications.
Presearch is one such organization that is using peer-to-peer networks to build a more transparent search engine platform. Additionally, the data management company, Yhat is working with Turo to use AI and peer-to-peer networks to power and improve their mobile applications.
30. Facial/Emotion Recognition:
Facial/Emotion detection and recognition is the process of identifying human emotions, especially verbal and facial expressions. The process uses artificial intelligence, among other techniques from multiple areas, like signal processing, machine learning, and computer vision, to get accurate results in real-time.
This technology is used by organizations to accurately study consumer behavior as well as to deliver desired services. Disney, Kellogg, and Unilever are the major brands that are highly invested in facial/emotion detection and recognition technology and are using it for market research, digital advertising, and one-on-one interview.
31. Image Recognition:
Powered by artificial intelligence, deep machine learning, and machine vision technology, Image Recognition is the ability of a software or machine to identify content, objects, people, and actions in images. This tech is mainly used in applications designed for factory automation, toll booth monitoring, and security surveillance.
Moreover, Qualcomm, NEC, Google, Microsoft, LTU Technologies, CatchoomTechnologies, Honeywell, Hitachi, and Apple are the key players investing significant resources and research into image recognition and related applications.
32. Marketing Automation:
The latest trend in the marketing industry, marketing automation is rapidly growing and is set to expand by 8.55% by the end of this year (2019), increasing the marketing capitalization to $5.5 billion.
It is utilizing artificial intelligence to better target customers, improve lead generation, as well as to increase its efficiency. Also, Market Automation is helping organizations streamline, automate and measure their marketing tasks and workflows to ensure customer engagement and retention. HubSpot, SendinBlue Email, Pardot, etc. are among the leaders in marketing automation.
33. Robots, Cobots, and Androids:
The advancement in artificial intelligence has led to the development of Robots, cobots, and androids that are becoming an integral part of various processes. Organizations around the world are investing excessive resources and money in the development of these software’s to further reap their benefits.
These are rapidly being incorporated in the consumer world in the form of vacuums, smart speakers, home automation tools, etc. and are being used to enhance and streamline corporate processes, manufacturing, shipping, and fulfillment. Companies like Amazon a key leader in the industry, as it is using robots, cobots, and androids to perform their day-to-day manufacturing processes.
34. Business Intelligence (Enhanced by AI):
Radical changes and advancements in predictive analytics, machine learning, and artificial intelligence (AI) have given way to a new generation of business intelligence (BI) tools that are leveraging the advantages of all these techs and enhancing their processes.
Business Intelligence (BI) is using AI to democratize data as well as to improve analytics adoption. Moreover, AI in BI is witnessed through AI-powered alerts, whether basic or advanced neural network alters, which are helping businesses stay in full control of their successful business factors. ThoughtSpot and Microsoft are two companies currently leading in the investment of AI and machine learning in business intelligence.
35. Human Botification:
In recent years, researchers are leveraging AI and machine learning to humanize machines and robots, which has enabled them to design an AI-powered conversational user experience that engages people on the veracity of the content.
This has given way to human botification, wherein bots are used to communicate with humans, to solve their queries, write content, among other things. Companies like LinkedIn, Facebook, and Google are already using this tech to botify human communication and to enable the exchange of structured and unstructured information naturally and efficiently.
36. Chatbot:
Chatbots are artificial intelligence computer program or software that simulates conversations with a user in natural language, either through the auditory or textual method. The AI-powered chatbots are designed to handle moderately sophisticated conversations.
Additionally, these leverage machine learning and natural language processing to understand the intent behind user’s requests, to account for their entire conversation history, as well as to respond to them in a human-like manner. Watson Assistant, Bold360, and Rulai are few of the most popular chatbots used around the world.
37. Autonomous Things:
Leveraging AI and its abilities, Autonomous Things or AUT takes the advanced machine learning a step further so that it can make complex decisions autonomously and automate functions that were initially performed by humans.
Build on the Internet of Things (IoT), it makes these seasoned decisions based on multiple factors about the current situation. Self-driving cars, drones, advanced robotics, etc. are all examples of autonomous things.
38. AI Optimized Hardware:
Artificial Intelligence (AI) and its rapid evolution have led to an explosion of new types of hardware powered by deep learning, machine learning, and other AI capabilities to power better and faster processes.
Hardware is being designed and optimized for AI workflow by major companies like IBM, NVIDIA, Google, and more with an aim to increase the performance and throughput of the systems, which will further speed up the training and enable the training of more complex models. the systems, which will further speed up the training and enable the training of more complex models.
39. Conversational AI-Powered Search:
The way people search the answer for queries and things online has changed drastically recently with the introduction of voice search. However, this has further reached new heights with the development of a conversational AI-powered search.
Embedded with Natural Language Processing and Understanding as well as machine learning, it will enable real-time search and will increase their extent to provide accurate results. WorkFusion, Conversica, Narrative Science, DigitalGenius, and Soundhound are some of the major companies leading in Conversational AI-Powered Search.
40. Embodied Agent:
Embodied Agents are another technology popular among organizations around the world. Also known as interface agents, embodied agents are intelligent agents that interact with humans and their environment through a physical body. Ananova and Microsoft Agents are two examples of embodied agents.
Moreover, Embodied Conversational Agents are the enhanced version of embodied agents that are usually computer generated and appear to be cartoon-like. They demonstrate properties similar to humans, including the ability to produce and respond to verbal and non-verbal communication.
41. Ensemble Learning:
An increasingly popular technique, ensemble learning tries to overcome some of the risks associated with single hypothesis algorithms. It is a powerful technique for improving the performance of a particular model.
By combining several methods it helps to improve the results of machine learning and enables the production of better predictive performance, as compared to a single model. Moreover, ensemble methods combine machine learning techniques into the predictive model to decrease bagging and boosting as well as to improve predictions or stacking
42. Evolutionary Algorithms:
A component of evolutionary computation, an evolutionary algorithm is a technology that has outperformed deep learning machines. It functions through the selection process in which the least fit members of the population are eliminated and the most fit are allowed to survive until a better solution is determined.
The goal of this technology is to create a computing code that solves a specific problem using an approach that is somewhat counterintuitive.
43. Friendly Artificial Intelligence:
A term coined by Eliezer Yudkowsky, friendly artificial intelligence or FAI is a hypothetical artificial general intelligence (AGI) or superintelligence that produces good, beneficial outcomes rather than harmful ones. It is a part of the ethics of AI and is related to machine ethics.
Moreover, the goal of a friendly AI is to stay aligned with the human’s goals and make things more benevolent rather than malevolent. It is, in short, duplicating, what we humans call common sense in order to stay aligned with our goals.
44. Intelligence Amplification:
Also known as cognitive augmentation and machine augmented intelligence, Intelligence Amplification (IA) is an effective use of information technology to augment, complement, and improve human intelligence. It is assumed that IA will help humans be more powerful than AI.
According to Michael Anissimov, the co-organizer of Singularity Summit, “the real objective of IA is to create super Einsteins, persons quantitatively smarter than any human being that has ever lived." Moreover, Microsoft is using artificial intelligence (AI) to boost intelligence amplification (IA) in their HoloLens to further enhance human creativity and
45. Neural Network:
Neural Networks or Artificial Neural Networks (ANN) is a set of algorithms designed and modeled after the human brain and is composed of artificial neurons or nodes. It uses artificial intelligence technologies like deep learning and machine learning to perform tasks like clustering, classifying and recognizing patterns.
The areas where ANN is currently being used include nonlinear system identification & control, game playing & decision making, pattern & sequence recognition, medical diagnosis, etc.
46. Neuro-Fuzzy:
Neuro-Fuzzy is a combination of fuzzy logic and artificial neural networks (ANN). It is used as a learning algorithm, inspired by neural network theory to determine its parameters.
The purpose of neuro-fuzzy is not limited to this, it is designed to solve the trade-off between neural networks' mapping precision and automation, as well as the interpretability of fuzzy systems.
47. Recursive Self-Improvement:
Associated with artificial intelligence (AI), recursive self-improvement is the self-improving software, that has the potential to develop superintelligence. The software is aware of its source code and has the ability to modify and create their own code in repeated cycles of improvement.
Seed AI is one such example that is designed for self-understanding, self-modification, and performing recursive self-improvement, with the assistance of which it develops greater intelligence at an exponential rate.
48. Self Replicating Systems:
Advancement in artificial intelligence has given way to self-replicating systems that are capable of reproducing itself autonomously, using deep learning and machine learning abilities.
Companies like Google have already had a breakthrough in the field, with AutoML machine-learning artificial intelligence software being able to replicate itself, without any human input or interference.
49. Sentiment Analysis:
Sentiment Analysis, which is also termed as opinion mining and emotion AI, is an automated process that is used to systematically understand, identify, assess, and analyze data and information collected from the masses.
With the recent progress in technology, machine learning techniques are expanding the possibilities of sentiment analysis. Moreover, Sentiment Analysis, along with machine learning, has become an integral part of AI and is using various tech like natural language processing, text analysis, computational linguistics & biometrics to systematically perform data analytics.
50. Strong Artificial Intelligence:
A type of artificial intelligence, Strong Artificial Intelligence is a constructor machine that simulates the human brain as well as their cognitive capabilities and functions. It is also known as Full AI and Artificial General Intelligence (AGI).
It enables machines and robots to mimic human abilities like self-awareness, problem-solving, reasoning, creativity, consciousness, sentience, and more. Furthermore, they are capable of interacting with their surroundings and learn from it, as well as picking up their own consciousness and language.
51. Superhuman:
Often used in the context of fictional characters, animals, and artificial intelligence, Superhuman is an entity or machine that surpasses the greatest human achievements, using human cognitive abilities.
IBM’s Deep Blue is one such example, as it defeated its human competitor, using reasoning, data analysis, and machine learning capabilities.
52. Swarm Intelligence:
Found throughout nature, like in ant colonies, swarm intelligence is recently being implemented into machines, robots, data mining, medicine and blockchain by researchers.
It mainly deals with natural and artificial systems that consist of many individual entities/machines/robots that coordinate with one another using decentralized control and self-organization. Researchers around the world are working to make it a common reality.
53. Symbolic Artificial Intelligence:
Symbolic Artificial Intelligence (AI), which is also known as Classical AI, is a term used for the collection of methods in artificial intelligence research that is concerned with attempting to explicitly represent human knowledge in a declarative form.
Symbolic AI or Good Old Fashioned Artificial Intelligence (GOFAI) as named by John Haugeland in his book Artificial Intelligence: The Very Idea (1985) as an approach is based on the assumption that many aspects of intelligence can be achieved by manipulating symbols.
54. Technological Singularity:
A term coined by John Von Neumann in 1958, the technological singularity defines the transformation resulting from an interaction between humans, technology, and artificial intelligence. It is a hypothetical scenario wherein artificial intelligence will rapidly improve orders of magnitude using techniques like recursive self-improvement.
This technology is thought to be beneficial for humans, as it could lead to fundamental changes and result in superintelligence, owing to its fast and powerful processing power.
55. Weak Artificial Intelligence:
Also known as Narrow AI, Weak Artificial Intelligence (AI) is concerned with one narrow task, that it is programmed to do. This type of AI is currently used in various software, applications, and products, such as Siri, Cortana, Alexa, and more.
Weak AI helps turn big data into usable information by detecting patterns and making useful predictions. Moreover, it simulates human cognition and benefits us by automating time-consuming tasks like performing data analysis.
56. Bot:
Bots are automated tools and software that perform simple and repetitive tasks, making them easier for humans. Also known as spiders, crawlers, and web bots, bots are mainly used as computer assistants to perform various tasks like indexing search engines as well as finding answers to human queries.
Companies like Skype, Google, Facebook, Apple, etc. are using Bots to improve user experience as well as to allow users to connect with them. Bots leverage natural language processing and understanding to perform tasks, which enables users to use natural language to get work done.
57. Blockhead:
Blockhead is a theoretical computer system that was invented as a thought experiment by Ned Block. This computer program has since made great advancement and is now capable of simulating artificial intelligence, by searching for things in a data repository.
With the assistance of this system, many tasks performed by artificial intelligence can be achieved, without any hassle, as it just requires a sufficient informative database.
58. Artificial Immune System:
Artificial Immune System (AIS) is a computational intelligence, rule-based, machine learning system that is inspired by the principles and processes of the immune system. This sub-field of biologically inspired computing is an effective tool for detecting anomalous behavior in the security domain of computing systems.
AIS comprises of two major types of research, the combination of mathematical and computational techniques for modeling of immunology, and the incorporation of immune systems processes for the development of engineering solutions.
59. Artificial Stupidity:
Artificial Stupidity, as suggested by its name, is an artificial intelligence that is intentionally dumbed, so as to make it more accessible, useful, and pleasing for users. It is a way of making superintelligent AI more human-like.
As it is possible that AI may pose an existential threat to humanity, with artificial stupidity, humanity can protect themselves from this threat by making AI more akin to human intelligence.
60. Humanized AI:
A type of Artificial Intelligence (AI), Humanized AI has the ability to understand and react to human emotions in a natural or human-like manner. It uses techniques like facial recognition, natural language processing, etc. to understand emotions like stress, anger, pain, etc. when displayed through speech, facial and physical expressions.
Researchers are working on humanized AI and are eager to implement it in sectors like healthcare, banking, financial services, and education, as it has the ability to not only derive insight from the user’s data but also to respond to them in a manner & language best suited to their emotional profile.
List of AI Projects:
“Man’s relationship with technology is complex. We always invent technology, but then technology comes back and reinvents us” ― Atul Jalan, Where Will Man Take Us?
Piloted by the breakthroughs in artificial intelligence technology, we are moving towards an advanced and autonomous world. Companies, both big and small, are already working on projects powered by artificial intelligence that will reshape industries as well as our society.
From IBM and Tesla to Google, Microsoft, and more the biggest names in the industry are working tirelessly to create a futuristic world and to keep up the momentum of technological progression.
As stated by Amy Stapleton, “We are entering a new world. The technologies of machine learning, speech recognition, and natural language understanding are reaching a nexus of capability. The end result is that we’ll soon have artificially intelligent assistants to help us in every aspect of our lives.”
Currently, artificial intelligence is not dominating the industry, processes, and technologies. However, with recent advancements and research, we are reaching there. AI, in short, has found its way into our daily lives in the form of virtual assistants like Alexa and Siri. And though it is not visible everywhere, AI is in the backdrop performing tasks to improve our day-to-day activities.
While the wait to see truly remarkable breakthroughs is still long, we can enjoy tantalizing glimpses of what is to come by going through various AI projects that are either on the verge of completion or are already on the way to reshape the future.
So, here is a list of some important projects that are using AI and its various technologies.
1. Blue Brain Project:
A Swiss brain research initiative led by the Founder & Director Professor Henry Markram, EPFL’s Blue Brain Project is an attempt to create an accurate, biologically detailed digital reconstruction and simulation of a rodent’s brain and ultimately the human brain, by the reverse-engineering mammalian brain to a molecular level.
2. Google Brain:
Initiated in 2011, Google Brain is a deep learning project part of Google X. The goal of the project is to create an artificial intelligence similar and equal to the human-level. The Google Brain Team is to improve machines' ability to hear and see so that they can better interact with humans.
3. Human Brain Project:
Launched in October 2013, by European Commission’s Future & Emerging Technologies (FET) scheme, Human Brain Project (HBP) is a 10-year scientific research project that enables researchers from all over Europe to practice and process advance knowledge in the field of neuroscience, computing, and brain-related medicine.
4. NuPIC:
NuPIC or Numenta Platform for Intelligent Computing is an open-source HTM implementation created by Numenta that allows algorithmic experimentation of its cortical algorithm.
5. 4CAPS:
Developed by Marcel A. Just at Carnegie Mellon University, 4CAPS or Cortical Capacity Constrained Concurrent Activation Based Production System is a cognitive architecture used to create computational models for cognitive neuroscience and more.
6. ACT-R:
Adaptive Control of Thought-Rational or ACT-R, as it is commonly known, is a cognitive architecture developed by John Robert Anderson and Christian Lebiere at Carnegie Mellon University. It is a theory of simulating and understanding human cognition and how people organize knowledge and produce intelligent behavior.
7. AIXI:
First proposed by Marcus Hutter in 2000, AIXI is a theoretical mathematical formalism for Artificial General Intelligence and is considered a reinforcement learning agent that maximizes the expected total rewards received from the environment. Though not computable, it combines Solomonoff Induction with sequential decision learning.
8. CALO:
Cognitive Assistant that Learns & Organizes or CALO is a cognitive project funded by DARPA. It attempts to integrate numerous AI technologies into a cognitive assistant or system that can reason, learn more experience, be told what to do, reflect on experiences, respond robustly, among other things.
9. CHREST:
Developed by Fernand Gobet at Brunet University & Peter C. Lane at the University of Hertfordshire, CHREST or Chunk Hierarchy & REtrieval STructures is a symbolic cognitive architecture based on the concepts of limited attention, limited short-term memories, and chunking. It closely simulated learning and models human perception, learning, memory, and problem-solving.
10. CLARION:
CLARION, which is an abbreviation of Connectionist Learning with Adaptive Rule Induction, is a computational cognitive architecture, developed under Ron Sun at Rensselaer Polytechnic Institute and the University of Missouri. It aims to investigate the fundamental structure of the human mind by synthesizing many intellectual ideas into a unified and coherent model of cognition.
11. CoJACK:
An extension to JACK, the multi-agent system or platform that adds cognitive architecture to the agents for eliciting more realistic or human-like behavior in the virtual environment. CoJACK is an ACT-R inspired extension that provides insights on behavior variability, situation awareness, and behavioral moderators.
12. Copycat:
Designed and developed by Douglas Hofstadter and Melanie Mitchell at the Indiana University Bloomington, Copycat is a model of analogy-making and human cognition. It is based on the concept of parallel terraced scan and is able to discover insightful analogies in a psychologically realistic manner.
13. DUAL:
DUAL is a general-purpose cognitive architecture that comprises of a unified description of mental representations, memory structure, and processing mechanism. Developed at the Central & Eastern European Center for Cognitive Science at New Bulgarian University, DUAL is a hybrid, multi-agent cognitive architecture that supports dynamic computation.
14. FORR:
Inspired by Herbert A. Simon’s idea of bounded rationality and satisficing, FORR was first developed in the City University of New York in 1990. It is a cognitive architecture that is used for learning and problem-solving.
15. IDA and LIDA:
Learning IDA or Learning Intelligent Distribution Agent is an integrated artificial cognitive system that attempts to model cognition in biological systems. Developed by Stan Franklin at the University of Memphis, this cognitive architecture is empirically grounded in cognitive science and cognitive neuroscience.
16. OpenCog:
An open-source software project that aims at creating an open-source framework for Artificial General Intelligence. Led by Ben Goertzel, OpenCog is a cognitive architecture that defines a set of interacting components designed to give rise to human-equivalent artificial general intelligence.
17. Procedural Reasoning System:
A popular agent architecture in artificial intelligence, Procedural Reasoning System (PRS) is a framework for constructing real-time reasoning systems that can perform complex tasks in a dynamic environment. Developed by Michael Georgeff and Amy L. Lansky at SRI International in 1980, PRS has inspired several works in AI, mainly in embedded and industrial applications.
18. Psi-Theory:
A systematic psychological theory, developed by Dietrich Dorner at the Otto-Friedrich University in Bamberg, Germany, Psi-Theory covers human action regulation, intention, and emotion. It models the human mind as an information processing agent, controlled by a set of basic physiological, social, and cognitive drives.
19. R-CAST:
Recognition Primed Decision-making enabled Collaborative Agents Stimulation Teamwork or R-CAST is a group decision support system based on research on naturalistic decision making. It is an agent architecture developed by a team led by Dr. John Yen at Pennsylvania State University. The R-CAST architecture is very flexible in that the architecture can be configured for a wide range of modeling purposes.
20. Soar:
A general cognitive architecture, Soar was originally created by John Laird, Allen Newell, & Paul Rosenbloom at Carnegie Mellon University. It integrates knowledge-intensive reasoning, reactive execution, planning and learning from experience, etc. to create a general computational system that has the same cognitive abilities as humans.
21. Society of Mind:
Born in discussions between Marvin Minsky and Seymour Papert in early 1970 at MIT Artificial Intelligence Lab, Society of Mind describes the collective behavior of simple and intelligent agents. Society of Mind Cognitive Architecture relies on a society of agents operating using metrics associated with the principles of artificial economics.
22. Subsumption Architectures:
A reactive robotic architecture, subsumption architecture is heavily associated with behavior-based robotics. The term was first introduced by Rodney Brooks and colleagues in 1986 and is now widely influential in autonomous robotics and other areas of AI.
23. AlphaGo:
Developed by Alphabet Inc’s Google Mind in London, AlphaGo is an AI agent that has the ability to create a learning algorithm, which it uses to beat human competitors in the Chinese strategy board Go. AphaGo had more powerful successors AlphaMaster (the version that defeated the world champion Go player), AlphaGo Zero, and AlphaZero.
24. Chinook:
Developed by a team led by Jonathan Schaeffer between 1989 to 2007 at the University of Alberta, Chinook is a computer program that plays English Draughts or checkers. It is the first to win the title of champion in the competition against humans.
25. Deep Blue:
Developed by IBM, Deep Blue is a chess-playing computer, popularly known for being the first-ever computer to defeat a human in the game of chess. Leveraging its machine learning and risk analysis capabilities, Deep Blue defeated world champion Garry Kasparov in 1997.
26. FreeHAL:
Though no longer active, FreeHAL is a self-learning chatbot that uses semantic network and technologies, like pattern recognition, stemming, part of speech databases, etc. to organize its knowledge to imitate human behavior within conversations.
27. Halite:
Created by Two Sigma, Halite is an open-source computer programming contest, wherein participants are asked to build bots on a two-dimensional virtual battlefield, in whichever language they choose.
28. Libratus:
Developed at Carnegie Mellon University, Pittsburgh, Libratus is the first artificial intelligence computer program, designed to play poker and that defeated world-class poker players in 2017. Creators of Libratus intend to generalize the AI for other applications.
29. Stockfish AI:
A free and open-source UCI chess engine, Stockfish AI is currently leading in many computer chess rankings and is available for various desktop and mobile versions. It is developed by a team of experts, Marco Costalba, Joona Kiiski, Gary Linscott, Tord Romstad, and other open-source developers.
30. TD-Gammon:
A computer backgammon program, TD-Gammon was developed in 1992 by Gerald Tesauro at IBM’s research center. It consists of a three-layer artificial neural network trained using a form of temporal difference learning technique, known as TD-lambda.
31. Operation Serenata de Amor:
Established in 2016 by data scientist Irio Musskopf, sociologist Eduardo Cuducos and entrepreneur Felipe Cabral, Serenata de Amor is an artificial intelligence cyber activism project used to analyze public expenditures and detect discrepancies in Brazil.
32. Briana:
Developed by Brainasoft, Briana is an intelligent personal assistant with a voice interface for Microsoft Windows OS. It uses a natural language interface and speech recognition to perform various tasks. Moreover, with time, it adapts the user's behavior to better anticipate needs.
33. Cyc:
World’s longest living Artificial Intelligence project, Cyc is an attempt to assemble a comprehensive ontology or database of everyday knowledge. Initiated by Douglas Lenat, it aims to enable AI applications to perform human-like reasoning.
34. Eurisko:
A language created by Douglas Lenat after being frustrated with the constraints of Automated Mathematicians to a single domain. Eurisko is used for problem-solving and consists of heuristics, including how to use and change its heuristics.
35. Google Now:
A feature of Google Search for Android & iOS Google apps and Chrome browsers, Google Now was used to deliver relevant information to users, based on their repeated actions, leveraging Google’s Knowledge Graph project. It was discontinued by Google in 2016 to give way to a better virtual assistance model, Google Assistant.
36. Holmes:
A backronym for Heuristics & Ontology-based Learning Machines & Experiential Systems, Holmes is a cognitive system developed by the Indian technology organization Wipro. Created by Ramprasad K.R., Holmes was finally announced in 2016 and is since used for the development of digital virtual agents, knowledge virtualization, predictive systems, etc.
37. Microsoft Cortana:
Created by Microsoft, Cortana is an intelligent personal virtual assistant, for Windows 10, Windows 10 Mobile, Windows Phone (8.1), Invoke smart speaker, Windows Mixed Reality, Amazon Alexa, etc. It uses natural language processing technology, indexed & stored user information to perform tasks like sets reminders, deliver appropriate results, etc.
38. MYCIN:
An early medical expert system or Artificial Intelligence (AI) program, MYCIN was used to treat blood infections. Written in it was developed in over five to six years in the 1970s at Stanford University. It attempted to diagnose patients based on reported symptoms and medical test results, using a simple inference engine and a knowledge base of 600 rules.
39. Open Mind Common Sense:
Founded in 1999, the Open Mind Common Sense (OMCS) is a freely available crowd-sourced knowledge base of natural language statements about the world. It is an artificial intelligence project, based at the Massachusetts Institute of Technology (MIT) Media Lab that aims to build and utilize a large commonsense knowledge base from the contribution of thousands of people worldwide.
40. P.A.N.:
A free text analyzer, also known as P.A.N. Poetry Analyzer or Textual Analyzer, P.A.N. allows users to analyze virtually any sort of text, be it fiction, poetry, history, newspaper, etc. Published by David Benjamin Bowes, this Java-based application uses conventional grammar rules to breakdown non-conventional texts and examine them for a different and new perspective.
41. Siri:
Released in October 2011, Siri is currently the most popular virtual assistance that is part of Apple Inc.’s Operating System for various devices. It is an intelligent personal assistant and knowledge navigator, with a voice interface for answering questions, making recommendations, etc. by delegating requests to a set of internet services.
42. SNePS:
Developed and maintained by Stuart C. Shapiro & colleagues at the State University of New York at Buffalo, SNePS is a knowledge representation and reasoning KRRA system that is simultaneously a logic-based, frame-based, and network based.
43. Viv:
An open AI platform, created by the developers of Siri and later acquired by Samsung Electronics (to know more read this article ) in October 2016, Viv enables developers to distribute their products through an intelligent and conversational interface.
44. Wolfram Alpha:
Released on May 18th 2009, Wolfram Alpha is a computational knowledge or answer engine that answers queries by computing the answer from externally sourced curated data. It was developed by Wolfram Alpha LLC, a subsidiary of Wolfram Research.
45. AIBO:
AIBO is an artificial intelligence Robotic Pet (explicitly Robotic Dog), designed and manufactured by Sony in their Computer Science Laboratory (CSL). It is meant to be a companion robot for adults that has the ability to create life-like expressions and a dynamic array of modements.
46. Cog:
Though now discontinued, Cog was a project developed by the Humanoid Robotics Group of the Massachusetts Institute of Technology to study cognitive science and AI. The idea behind creating Cog was the hypothesis that humanoid intelligence requires humanoid interactions with the world, like infants.
47. Melomics:
Founded by Francisco Vico and launched in 2012, Melcomics is a computational system used for the composition and synthesization of music, without any human intervention.
48. AIML:
An Artificial Markup Language, developed by the Alicebot free software community and Dr. Richard S. Wallace, during 1995-2000, AIML is an XML dialect used for creating natural language software agents.
49. Apache Lucene:
Written by Doug Cutting and initially released in 1999, Apache Lucene is a free, open-source, high performance, and full-featured text search engine library entirely in Java. It is a technology suitable for nearly any application that requires full-text search, especially cross-platform.
50. Apache OpenNLP:
Developed by the Apache Software Foundation, Apache OpenNLP library is a machine learning based toolkit used for processing natural language text and to support the most common NLP tasks, like sentence segmentation, tokenization, language detection, part-of-speech tagging, etc.
51. Artificial Linguistic Internet Computer Entity (A.L.I.C.E.):
Also known as Alicebot or Alice, A.L.I.C.E. is an award-winning natural language processing chatterbot, inspired by Joseph Weizenbaum's ELIZA program. Originally composed by Richard Wallace, this free software chatbot is created in Artificial Intelligence Markup Language (AIML).
52. Cleverbot:
An evolved version of Jabberwacky, Cleverbot is a chatterbot web application, also available as an iOS, Android, & Windows Phone app. Created by Rollo Carpenter, a British AI scientist, Cleverbot uses an artificial intelligence algorithm to have conversation with humans.
53. ELIZA:
Created by Joseph Weizenbaum at the MIT Artificial Intelligence Laboratory from 1964 to 1966, ELIZA is an early natural language processing computer program used to demonstrate the superficiality of communication between humans and machines, and to parody person-centered therapy.
54. Jabberwacky:
A chatterbot created by Rollo Carpenter, the British AI scientist & programmer, to mimic human interactions and simulate natural human chat, in an interesting, entertaining, and humorous manner. Jabberwacky was an early attempt of Carpenter to create artificial intelligence through human interaction.
55. Mycroft:
World’s first open-source and free intelligent personal voice assistant for Linux-based Operating Systems, Mycroft uses a natural language user interface to perform requested tasks seamlessly. Written in Python, it is developed by the Mycroft AI team, including Ryan Sipes and Joshua Montgomery.
56. PARRY:
It is an early example of a chatterbot, written and implemented by psychiatrist Kenneth Colby, at Stanford University, in the year 1972. This chatterbot, like ELIZA, aimed at simulating humans with paranoid schizophrenia.
57. SHRDLU:
Written in Micro Planner, Lisp programming language, SHRDLU is a natural language processing computer program developed from 1968 to 1970 by Terry Winograd at the MIT Artificial Intelligence Laboratory. This program uses AI to interpret the natural language of a human user, who issues commands to manipulate a virtual world.
58. SYSTRAN:
SYSTRAN is a machine translation technology designed by the company of the same name, founded by Dr. Peter Toma in 1968. It is used for real-time language translation solutions by various high-scale organizations like Yahoo!, AltaVista, Google (Until 2007), among others, to boost collaborations.
59. 1 The Road:
1 The Road is an experiment novel composed by AI (Car), with an aim to emulate Jack Kerouac's On the Road. and published by Jean Boîte Éditions in 2018. Ross Goodwin, a prominent Artificial Intelligence creator, outfitted a car with a laptop and various sensors, whose output was turned into words by the AI and later turned into a manuscript.
60. SEAS:
Synthetic Environment for Analysis & Simulations, SEAS is a model of real world used to try out models or techniques in a publicly known, realistically detailed environment. Developed at Purdue University, this model uses simulation and AI to predict & evaluate future events and course of actions and is currently used by Homeland Security, the United States Department of Defense, & among others.
61. Apache Mahout:
Designed and developed by the Apache Software Foundation, Apache Mahout is used to produce free implementations of distributed or scalable machine learning algorithms focused on collaborative filtering, clustering, and classifications.
62. Deeplearning4j:
Deeplearning4j is an open-source, distributed, deep learning programming library, written for Java, Java Virtual Machine (JVM), and Scala. Integrated with Hadoop and Apache Spark, this framework is developed by a machine learning group led by Adam Gibson, with headquarters in San Francisco and Tokyo.
63. H2O:
Produced by the company H2O.ai, H2O is a leading open-source, data science, and machine learning platform that aims at developing an analytical interface for Cloud Computing, providing users with tools for data analysis.
64. Keras:
Authored and maintained by François Chollet, a Google engineer, Keras is an open-source neural network API written in Python. This high-level neural-network library enables fast experimentation with deep neural networks and is designed to be user-friendly, modular, and extensible. Moreover, it is capable of running on TensorFlow, CNTK, or Theano.
65. Microsoft Cognitive Toolkit:
Microsoft Cognitive Toolkit, previously known as CNTK and usually referred to as The Microsoft Cognitive Toolkit, is an open-source toolkit, developed by Microsoft Research, for commercial grade distributed deep learning and for building artificial neural networks.
66. OpenNN:
OpenNN or Open Neural Networks Library is an open-source software library, written in the C++ programming language for implementing neural networks. Developed by Artelnics, it contains sophisticated data mining algorithms to deal with various machine learning solutions.
67. PyTorch:
Primarily created by Facebook’s artificial intelligence research group, PyTorch is a free and open-source Tensor & Dynamic neural network in Python. It is a deep learning library, released under the Modified BSD license.
68. TensorFlow:
Developed by the Google Brain Team for both research and production, TensorFlow is a free & open-source software library for machine learning. Released under the Apache license, on November 2019, it has a comprehensive, flexible ecosystem of tools, libraries, and more.
69. Theano:
An open-source project, Theano is a Python library for defining, optimizing, manipulating, and evaluating mathematical expressions, including matrix-valued ones. It was initially released in 2007 by the Montreal Institute for Learning Algorithms (MILA) at the University of Montreal.
70. Neural Designer:
A machine learning software, written in C++, Neural Designer is a software tool for data analytics based on neural networks. It contains a graphical user interface that simplifies data entry & interpretation of results. Moreover, it allows the rapid and effective development of machine learning projects.
71. Neuroph:
An open-source, object-oriented Artificial Neural Network Framework, Neuroph is written in Java and hosted at SourceForge under the Apache License. It provides Java class library and GUI tool, which can be used to create and train neural networks.
72. OpenCog Prime:
Also known as primeAGI, OpenCog Prime is an architecture for robot & virtual embodied cognition, used to create powerful and human-equivalent artificial general intelligence by implementing it with OpenCog, a project that strives to build an open-source artificial intelligence framework and is used by companies like Cisco, Huawei, among others.
73. RapidMiner:
Now developed commercially, on an open core model, by the company of the same name, RapidMiner is an integrated data science software platform for data preparation, machine learning, data mining, deep learning, and predictive analytics.
74. Weka:
Developed at the University of Waikato New Zealand, Weka or Waikato Environment for Knowledge Analysis is open-source software written in Java. Moreover, it is a collection of machine learning algorithms for data mining tasks and contains tools for data preparation, classification, regression, clustering, etc.
75. Data Applied:
Developed by the company of the same name, Data Applied implements a collection of visualization tools and algorithms for data analysis & data mining. It also supports various other ganalytical tasks like visual reporting, time series forecasting, clustering, etc.
76. Grok:
The first commercial product of Numenta, the machine intelligence company founded by Jeff Hawkins, Grok offers anomaly detection for IT analytics and provides an insight into IT systems to identify behavior & reduce business downtime.
77. Watson:
A computer system capable of answering questions posed in natural language, Waston was developed by a research team led by Devid Ferrucci in IBM’s DeepQA Project. Though initially developed to answer questions on the quiz show Jeopardy, Watson is now used to uncover and share data-driven insights and to spur cognitive applications.
78. DEAP:
A novel computation framework for rapid prototyping and testing of ideas, developed at the Universite Laval in 2009, Distributed Evolutionary Algorithms in Python or DEAP incorporates the data structures and tools required to implement common evolutionary computation techniques.
79. Chainer:
An open-source deep learning, flexible, and intuitive framework written in Python and developed by Japanese venture company Preferred Networks in partnership with IBM, Intel, Microsoft, & Nvidia, Chainer provides automatic differentiation and object oriented high level APIs to build and train neural networks.
80. Gensim:
Implemented in Python and Cython, Gensim is an open-source library for topic modelling, document indexing, natural language processing, and similarity retrieval using machine learning.
81. Neon:
Discontinued in 2018, Neon is a deep learning framework created by Nervana Systems to deliver industry-leading performance. This open-source framework helped integrate visualizations with data and was designed for ease of use and extensibility.
82. Nilearn:
Developed by a community of people including INRIA Parietal Project Team and the scikit learn team, Nilearn is a Python module for quick & statistical learning on NeuroImaging data. It uses the scikit learn Python toolbox for multivariate statistics with applications.
83. scikit-learn:
Started as scikits.learn, scikit-learn is a Google Summer of Code project by David Cournapeau. It is a free software machine learning library for Python that features various classification, regression, and clustering algorithms.
84. CAFFE:
Developed at the University of California, Berkeley, CAFFE or Convolutional Architecture for Fast Feature Embedding, is an open source deep learning framework written in C++, with a Python interface.
85. StatsModels:
A free software released under the BSD license and build on top of numerical libraries NumPy and SciPy, StatsModel is a Python package, which is used to explore data, estimate statistical models and perform statistical tests.
86. Pylearn2:
Designed to make machine learning research easy as well as for flexibility and extensibility, Pylearn2 is a machine learning library, with most of its functionality built on Theano. It is released under BSD (clause 3) license and is used mainly for commercial purposes.
87. PyMC:
Initially released under the Academic Free Licence, PyMC is a Python module used for implementing statistical models and fitting algorithms. It is flexible and extensible, which makes it suitable for large suite of problems.
88. Orange3:
The third version of Orange, the open-source data visualization, machine learning, and data mining toolkit, Orange3 was released under the GPL in 2016. Complete revamp of Orange, it has a NumPy data model, Python-based data analytics compatibility, etc.
89. Annoy:
Built by Erik Bernhardsson, Annoy or Approximate Nearest Neighbour Oh Yeah is a C++ library with Python bindings. It is an open-source library that is used to search for points in space that are close to a given query point.
90. PyBrain:
A modular Machine Learning library, PyBrain is an acronym of Python-Based Reinforcement Learning, Artificial Intelligence, & Neural Network Library. It offers users flexible, powerful, and easy-to-use algorithms for Machine Learning and a predefined environment to test and compare algorithms.
91. MXNet:
Designed to be distributed on dynamic Cloud infrastructure by Apache Software Foundation, MXNet is an open-source deep learning software framework, used to train and deploy deep neural networks. Supported by public Cloud providers AWS and Microsoft Azure, the MXNet library is portable and can scale to multiple GPUs & machines.
92. Magenta:
Powered by TensorFlow and distributed as an open-source Python Library, Magenta is a research project started by researchers and engineers from Google Brain Team, with contributions from other stakeholders. It explores the role of machine learning in the process of creating art and music.
93. Style2Paints:
Also known as PaintsTransfer, Style2Paints is an Artificial Intelligence for animation colorization. It performs the task by combining an enhanced residual U-net generator and an auxiliary classifier discriminator as adversarial network.
94. Deep Voice Conversion:
Developed by Dabi Ahn, Deep Voice Conversion is a project started with the goal to convert an individual’s voice to a specific target voice. It is a many-to-one voice conversion system that uses deep neural networks for conversions.
Complete List 80+ AI Projects based on a different category.
Project Name | Type |
---|---|
Blue Brain Project | Brain-Inspired |
Google Brain | Brain-Inspired |
Human Brain Project | Brain-Inspired |
NuPIC | Brain-Inspired |
4CAPS | Cognitive Architectures |
ACT-R | Cognitive Architectures |
AIXI | Cognitive Architectures |
CALO | Cognitive Architectures |
CHREST | Cognitive Architectures |
CLARION | Cognitive Architectures |
CoJACK | Cognitive Architectures |
Copycat | Cognitive Architectures |
DUAL | Cognitive Architectures |
FORR | Cognitive Architectures |
IDA and LIDA | Cognitive Architectures |
OpenCog | Cognitive Architectures |
Procedural Reasoning System | Cognitive Architectures |
Psi-Theory | Cognitive Architectures |
R-CAST | Cognitive Architectures |
Soar | Cognitive Architectures |
Society of mind | Cognitive Architectures |
Subsumption architectures | Cognitive Architectures |
AlphaGo | Games |
Chinook | Games |
Deep Blue | Games |
FreeHAL | Games |
Halite | Games |
Libratus | Games |
Stockfish AI | Games |
TD-Gammon | Games |
Serenata de Amor | Internet activism |
Braina | Knowledge and reasoning |
Cyc | Knowledge and reasoning |
Eurisko | Knowledge and reasoning |
Google Now | Knowledge and reasoning |
Holmes | Knowledge and reasoning |
Microsoft Cortana | Knowledge and reasoning |
Mycin | Knowledge and reasoning |
Open Mind Common Sense | Knowledge and reasoning |
P.A.N | Knowledge and reasoning |
Siri | Knowledge and reasoning |
SNePS | Knowledge and reasoning |
Viv (software) | Knowledge and reasoning |
Wolfram Alpha | Knowledge and reasoning |
AIBO | Knowledge and reasoning |
Cog | Knowledge and reasoning |
Melomics | Music |
AIML | Natural language processing |
Apache Lucene | Natural language processing |
Apache OpenNLP | Natural language processing |
Artificial Linguistic Internet Computer Entity | Natural language processing |
Cleverbot | Natural language processing |
ELIZA | Natural language processing |
Jabberwacky | Natural language processing |
Mycroft | Natural language processing |
PARRY | Natural language processing |
SHRDLU | Natural language processing |
SYSTRAN | Natural language processing |
the road | Other |
synthetic environment for analysis and simulations | Other |
Multipurpose Projects | |
Mahout | Software Libraries |
Deeplearning4j | Software Libraries |
H2O | Software Libraries |
Keras | Software Libraries |
Microsoft Cognitive Toolkit | Software Libraries |
OpenNN | Software Libraries |
PyTorch | Software Libraries |
TensorFlow | Software Libraries |
Theano | Software Libraries |
Neural Designer | GUI Frameworks |
Neuroph | GUI Frameworks |
OpenCog | GUI Frameworks |
RapidMiner | GUI Frameworks |
Weka | GUI Frameworks |
Data Applied | Cloud Services |
Grok | Cloud Services |
Watson | Cloud Services |
DEAP | Open Source |
Chainer | Open Source |
Gensim | Open Source |
neon | Open Source |
nilearn | Open Source |
scikit learn | Open Source |
caffe | Open Source |
statsmodel | Open Source |
pylearn2 | Open Source |
pymc | Open Source |
orange3 | Open Source |
Annoy (Approximate Nearest Neighbour Oh Yeah) | Open Source |
pybrain | Open Source |
Fuel | Open Source |
Mxnet | Open Source |
magenta | Open Source |
style2paints | Open Source |
Deep voice conversion | Open Source |
Other Examples of Artificial Intelligence(AI) :
In the preceding section, we introduced a list of 80+ AI-based projects, formulated because of the tremendous success Artificial Intelligence and its branches have achieved as well as the potential they offer. It indicates that Artificial Intelligence is no longer just a mere theory but has found its foothold in every industry and sector, and is going to continue growing irrespective of the threat or concern theorists and researchers have associated with it.
With us moving closer to 2020, it is no wonder to see AI transcend from the lab to offices, hospitals, construction sites, factories, and being a part of consumer’s life. In short, the impact of AI and the possibilities it offers is nowhere near, and it is bound to increase in the coming years.
Some of the other examples where this impact will be most evident are:
1. Voice Recognition:
Alternatively known as Speech Recognition, Voice Recognition is the ability of a machine or program to receive, interpret, understand, and provide results to spoken commands. It is commonly used to operate a device, perform commands, or write through verbal instructions. It is now being used predominantly in various Virtual Assistants like Siri, Alexa, etc. with the help of machine learning and natural language processing techniques.
2. Customer Experience:
From data unification, business contest, chatbots & virtual assistants, to predictive personalization, real-time insights delivery, etc. organizations are leveraging AI’s machine learning, natural language understanding, and natural language processing capabilities to understand, collect, and analyze customer sentiment and feedback to deliver them the best customer experience, which in-process delivers improved customer satisfaction and loyalty.
3. Decision Making:
Among the many possibilities that Artificial Intelligence offers, improved decision making is the most prominent one. It has enabled organizations to get real-time data through means like coordinated data delivery, data trends assessment, forecasting, data consistency development, anticipating user data needs, bots, forms, and more, which together help them make more informed and useful decisions.
4. Pattern Recognition:
Pattern Recognition is another area powered by machine learning and its components. Closely akin to artificial intelligence and machine intelligence, Pattern Recognition, like voice recognition is the automated recognition of patterns and regularities in data, through the use of computer algorithms, which further uses regularities to classify the data into different categories. This classification is based on two factors, the knowledge already gained from patterns &/or their representations or through statistical information extracted from the same.
5. Forecasting:
Just as AI is pervading many aspects of our lives, similarly it is revolutionizing Forecasting with the assistance of various techniques like Artificial Neural Networks, Data Mining, Machine Learning, Pattern Recognition, and Support-Vector Machines, which help it outperform human capabilities. Forecasting, which is the process of making predictions of the future based on past and present data as well as through trend analysis, will soon be able to fix perennial blind spots and be more accurate than humans can ever be.
6. Assessment:
The use of Artificial Intelligence in Assessment has ramped up in recent years, with it driving digital transformation outcomes for enterprises. AI is being used along with Robotic Process Automation, Machine Learning, Pattern Matching, & Natural Language Processing to analyze and interpret results and vast amounts of data and improve and simplify the process of assessment.
7. Research:
A wave of the future, AI is transforming the way researchers and inventors perform research, as it has accelerated the process and simplified how they discover, access, and apply data to develop a new product or process. From extracting patterns and insights from data to processing and analyzing it, Artificial Intelligence has made every step of research and development quick, simple, and effective, with the help of various technologies like data mining, machine learning, natural language processing, etc.
8. Event Processing:
Event Processing is a methodology of tracking and analyzing the stream of events to extract meaningful insights into real-world events. It further comprises of two segments, Event Stream Processing, and Complex Event Processing (CEP), which are further used in the process. However, nowadays, organizations are using machine learning and analytics models to make event processing proactive and to calculate predictions regarding events in real-time. Some of the Machine Learning techniques used by organizations for prediction purposes are logistic regression, linear regression, K-Means clusterings, etc.
9. Productivity:
The idea of AI as a productivity solution has been in conception for years, and now it has found its stronghold in various industries. As predicted by Accenture, by 2023, AI will have the power to increase productivity by 40% or more, and considering the way companies are leveraging artificial intelligence and its technologies, there is no doubt that this won’t be a reality. AI has enabled companies to produce quality products faster and with more efficiency and offered various other benefits, like accurate demand forecasting, predictive maintenance, hyper-personalized manufacturing, optimizing manufacturing processes, automated material procurement, etc.
10. Design & Design Tools:
The growing impact of AI is evident everywhere, and the field of designing is no exception. However, the role of AI and AI-based design tools is not to replace humans but to assist human creativity. AI algorithms have already usurped the traditional way of designing, and one such example is enabling designers to generate millions of unique designs for Nutella. In short, AI in designing is mostly about speed, optimization, and efficiency and helping designers create unique products and their versions while maintaining the brand style and design integrity.
11. Efficiency:
With 2020 just few months away, theorists are clear about the area where artificial intelligence has had the greatest impact, irrespective of the industry or the sector. Organizations worldwide are leveraging machine learning, automation, data analytics, and other technologies to improve the business efficiency. These techniques have helped businesses assess great amount of data and get an accurate results, which enable them to make better decisions. That’s not all. AI, with machine learning and automation is increasing sales, improving User Experience, expanding business operations, streamlining in-house processes, providing quality control, etc.
12. Human-Computer Interaction:
Since their introduction, the level of interaction between humans and computers is limited to keyboards, mouse, touchscreen, etc. It is now that artificial intelligence and Human-Computer Interaction (HCI) are converging that various technologies are being used to change that. AI is powering speech-based and gesture-based systems with natural language processing, computer vision, neurotechnology, etc. to fundamentally change human computer interaction from simple, unimodal channels to complex, multimodal channels like verbal and non-verbal signals.
13. Games:
Ever since the introduction of artificial intelligence, it has been an integral part of the gaming industry. Be it the first computer chess, Deep Blue to defeat the human champion in 1997 or the latest video games developed with responsive, adaptive, and intelligent behaviors, similar to human-like intelligence, the role of AI is expanding greatly in the Gaming Industry with every year.
14. Problem Solving:
With business processes becoming complex and complicated day by day, the need for quick and effective problem-solving process is increasing swiftly. It is with the help of artificial intelligence that organizations are able to achieve this by combining it with predictive analysis applied to the problem-solving process, wherein the developed machine learning algorithm is used to compare incidents, data, and other provided past details to suggest root causes, corrective actions, and other necessary details, for a quick and efficient solving.
15. Resilience:
The field where the implication of artificial intelligence is most prominent and benefiting is in resilience and disaster management. Organizations, both private and official are working with Artificial Intelligence, Big Data & Analytics, and machine learning technologies to create systems that process large scale crisis data to gain an insight into emergencies, detect and predict how they develop, analyze their impact, and offer assistance in disaster response and resource allocation. AI, in short, may soon help countries better prepare for emergencies, save lives, limit economic impact, improve real-time communication during disasters, among other things.
